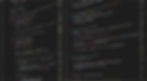
Despite its failings, Artificial Intelligence (AI) programs are quickly becoming an essential part of many industries, especially financial services like lending, where large amounts of data need to be processed quickly and fairness is crucial.
Yet AI often amplifies and perpetuates human racial biases. In 2018, MIT researcher Joy Buolamwini proved facial recognition programs are racist. A 2021 investigation by The Associated Press and The Markup found lenders using mortgage approval algorithms were 80% more likely to turn down Black applicants and 40% more likely to reject Latino applicants, in comparison with white applicants. A 2019 study by the University of California at Berkeley researchers also found that Black and Latino applicants were charged higher mortgage interest rates and refinancing fees.
While AI bias affects all industries, its existence in algorithms used by finance and lending organizations continues the tradition of reducing wealth in marginalized communities. When communities of color are denied loans, or offered loans at higher interest rates, they end up paying a higher premium for access to the same financial opportunities white people can easily access. This inequity widens the racial wealth gap further.
This bias can “exacerbate and worsen the minority status of minorities,” says Russel Geronimo Stanley, a Philippine-based lawyer who is conducting research examining bias and prejudice in AI-based financial services algorithms. “So if under a conventional financial system, they were already disadvantaged and underprivileged, [AI bias] will be worsening their situation.”
“Most financial services companies have what we call facially neutral policies. They do not intend to discriminate against minority groups, [but unintentional bias can occur], based on the way their algorithms and AI processes are designed,” he adds.
There are two kinds of AI-based algorithms: supervised and unsupervised. Supervised AI works by learning from clearly labeled datasets, while unsupervised AI learns and draws its own conclusions from data. Think of it as a child learning in a classroom versus being left alone to learn on her own.
The algorithms used in lending and finance are usually of the unsupervised kind. Unsupervised AI and machine-learning algorithms work by examining large sets of data and identifying patterns linking variables such as age, profession, internet usage habits, and other characteristics with creditworthiness.
There is little to no human intervention involved, and in most cases, one cannot tell which variables the algorithm is considering to draw its conclusions. It could be variables traditionally used to predict creditworthiness such as education, profession, or address—or it could be something entirely unrelated, such as preferred cuisine or the frequency of posting on Instagram.
“The algorithm looks at more variables than a human can, and finds correlations with credit behavior,” Stanley says. “Let's say a certain person or group of people love a particular cuisine. Nothing to do with lending, if you think about it. But for some reason, there is a kind of correlation with their credit behavior or performance. The algorithm can detect correlations where human beings are unable to detect, so that's where the bias is coming from.”
The use of AI in financial services, or any industry where numerous applications need to be processed quickly, is inevitable—and becoming the norm. But using AI doesn’t mean accepting biased and prejudiced algorithms. The path to identifying and rooting out these biases can broadly be classified three ways:
Ensuring a fair and equitable dataset;
Examining the algorithms and rules that drive the systems; and
Using disparate impact tests to seek out bias.
The first of these involves closely examining and rethinking the datasets AI algorithms are trained on. When programs receive biased or inequitable data, they inherit programmed bias and perpetuate the same racial discrimination of their human predecessors.
“If you're going to feed credit data, based on a data set that is already no longer a representative sample of the population, then naturally the AI will turn out garbage, inequitable, unfair results,” Stanley says. “We need to clean up the datasets in such a way that they are representative of the population.”
It’s also important to examine the algorithms themselves, especially when it comes to the selection of the variables that determine creditworthiness. Rather than focusing on immovable variables, such as education, address, profession, or religion; dynamic variables like utility payment history, credit card bills, and insurance premiums can be assigned a higher weightage.
This puts traditionally underserved communities on a more equal footing.
“AI bias is not a single source problem,” Stanley says. “The most urgent thing right now is [for consumer regulatory boards to] pass a regulation, or administrative issuance requiring disparate impact tests for algorithms [used in the finance and lending industry].”
The emphasis on requiring disparate impact—a way to examine the output of a particular program and compare its impact on a privileged group to its impact on a non-privileged group—tests for AI programs can reduce bias and ensure they meet a certain baseline of fairness. Many federal laws in the U.S. have disparate impact provisions, and AI-based programs could benefit from this approach.
Even in the absence of legislation, organizations that wish to avoid discriminatory bias in their systems can use disparate impact analyses and tests to evaluate their algorithms and processes. Any organization, especially in the financial sector, that uses AI programs has a responsibility to regularly examine their systems, eliminate bias, and ensure their systems are truly equitable and fair. Otherwise, any declarations of their commitment to diversity are just hollow promises.
Author Bio: Aishwarya Jagani is an independent journalist whose work examines the human impact of technology. Her work has appeared in BBC, Wilson Quarterly, The Open Notebook, Index on Censorship, Scroll, The Quint and other publications.